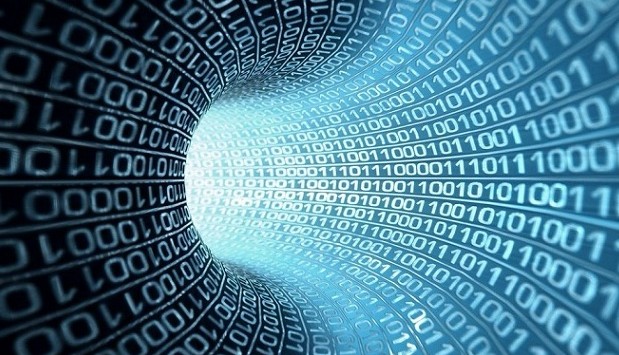
Can a machine read company announcements?
Regular readers will know that our investment process takes advantage of quantitative analysis to identify potentially interesting investment opportunities. To do this analysis, we “train” a computer model to distinguish between strong and weak investment candidates using historical data, and then apply the resulting model to try to try to identify tomorrow’s successful investments.
As with any investment approach, there are no guarantees of short term success, but our analysis leads us to think that this approach can add considerable value over longer stretches of time.
The historical data we use tends to be hard numbers, for example: fundamental ratios extracted from company financials, broker consensus earnings forecasts, and market trading data. However, a lot of the company information available to us is in text format – the contents of company announcements, annual reports and the like.
So, is there scope to train a machine to extract insights from this type of text data as well as the hard numbers? Analysis of text is a well-established part of the broader field of machine learning, and given the large volumes of company announcements and commentary released, it is tempting to think that a machine might be able to help with the grunt work involved in covering it all.
With this in mind, we spent some time over the Summer holidays gathering large volumes of ASX company announcements and reports, matching these with subsequent investment performance and testing different techniques for slicing the information up, to see what the machines could make of it.
I should note that we also spent some part of the Summer holidays at the beach. All things in moderation.
There are many ways to analyse this sort of data, but common approaches include examining the frequency of particular words or word combinations, assigning scores to words to capture (for example) their degree of optimism or pessimism, and analysing the overall style of the document through sentence length and word syllable count. For anyone interested in learning more, the following paper provides a pretty good survey of techniques and recent academic research as it applies to finance: Textual Analysis in Accounting and Finance: A Survey
This field of enquiry feels like it should have some promise, and our own analysis of ASX company announcements and reports yielded a few tantalising slivers of potential value, but on close examination those slivers appear to be quite thin, and probably not enough to warrant modification to our existing quantitative models. It may well be that more value can be extracted with further refinement of these techniques, but at this stage the results are a bit lukewarm.
One of the important challenges seems to be that written language is far less precise than numerical data. For example, there can be little doubt about which of two companies offers the best ratio of last year’s dividend to today’s share price, but when the CEOs use different adjectives to describe operational performance, comparisons become tricky.
One interesting observation we did make is that companies that have made larger numbers of price sensitive announcements appear to perform slightly worse than companies that have made fewer price sensitive announcements. This makes some intuitive sense, as a CEO who wishes to support his share price (ahead of a takeover, equity raise or personal share sale perhaps) can do so for a period of time by putting out as much good news as possible.
If nothing else, this serves as a reminder that in interpreting any piece of communication, it pays to be aware of the possible agenda behind it, as well as the content of the communication itself.
Dennis
:
Based on the above post I imagine the team at RM have read Investing Between the Lines by L.J. Rittenhouse. Recommended by Warren Buffett in one of his annual shareholder letters. Good book. I enjoyed it.
Joe
:
What happens if the intelligent agent is able to predict the companies that will succeed in our future world better than any human investor. Evidence of this is demonstrated by IBM’s Watson which produced a cancer fighting protocol for a patient that even the best medical specialists could not do.
What happens to the zero-sum game we play? Or do you believe it’s possible that, once all investors learn about the capabilities of these AI, only the most efficient companies survive and investing, as we currently know it, will become apart of our history?
Tim Kelley
:
While machines will take over in many fields, I suspect in financial markets it is not enough for machines to be better than humans to fully displace them. They would need to be perfect. Consider this: if trading is dominated by machines then share prices will reflect the collective insight of all the machines. All a human investor needs is a single relevant fact or insight not accounted for by the machines, and they would be able to exploit this by trading at the price the machines collectively deem “fair”.
Joe
:
Hi Tim,
Hi Tim,
This is one area of investing I am particularly interested in . I have reviewed several papers on the best formula based methodologies to successfully invest in shares, some of which I use but without an automatic protocol are difficult to implement e.g. Piotroski F-score method, Estimated Rev increase up 5%, O’Neils CAN SLIM etc. Despite academic research showing the success of these methods, there are surprisingly few funds adopting these methods, probably because of the difficulty in their implementation.
With today’s AI being able to read & interpret financial reports better & with deep learning algorithms using neural networks (like those in the game GO) do you think that the market will stop being, as Ben Graham calls it, a voting machine? i.e. if AI can predict with greater accuracy how a company performs (even 80% of the time) then why would you bother investing any other way? The risk would barely be worth it.
I realize this is just the beginning of “Intelligent” AI delving into this area but once it takes a hold (I expect with the next 5 years), what would you predict to be the outcome for your ordinary investor & for the industry as a whole?
Regards,
Joe
Tim Kelley
:
An interesting topic, Joe. I think that machines will certainly have a growing role in making investment decisions as the technology improves and becomes better-accepted. However, one of the critical factors for investment success is a point of difference – you can’t beat the market by doing what the rest of the market does. I think this places a limit on the “market share” the machines will be able to take, and ensures that there will be room for skilled human investors.